Policy Architectures
Contents
Policy Architectures#
We provide three vision-language models for encoding spatial-temporal information in robot learning for LLDM.
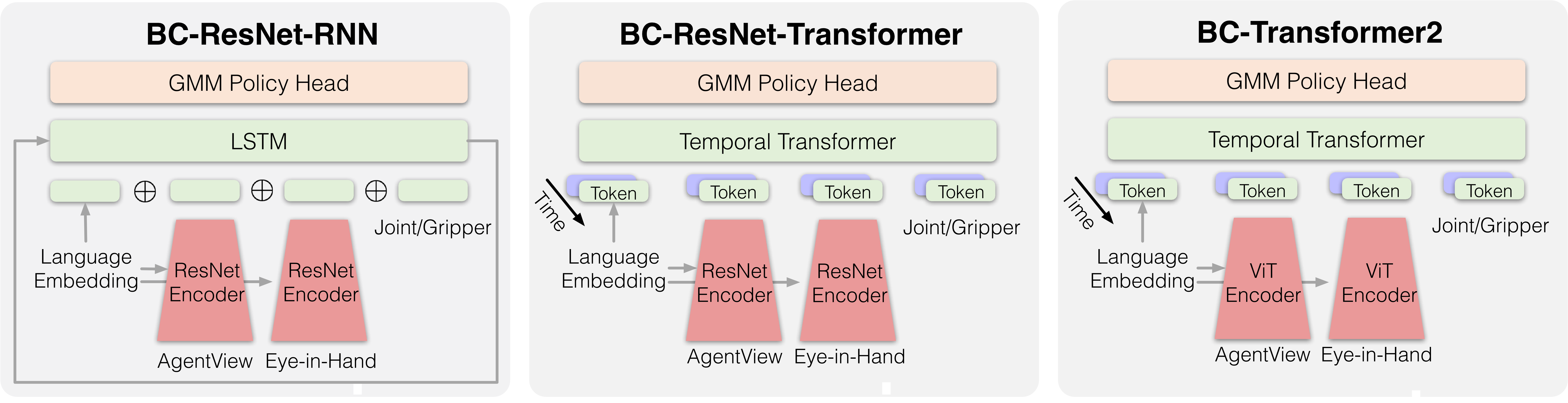
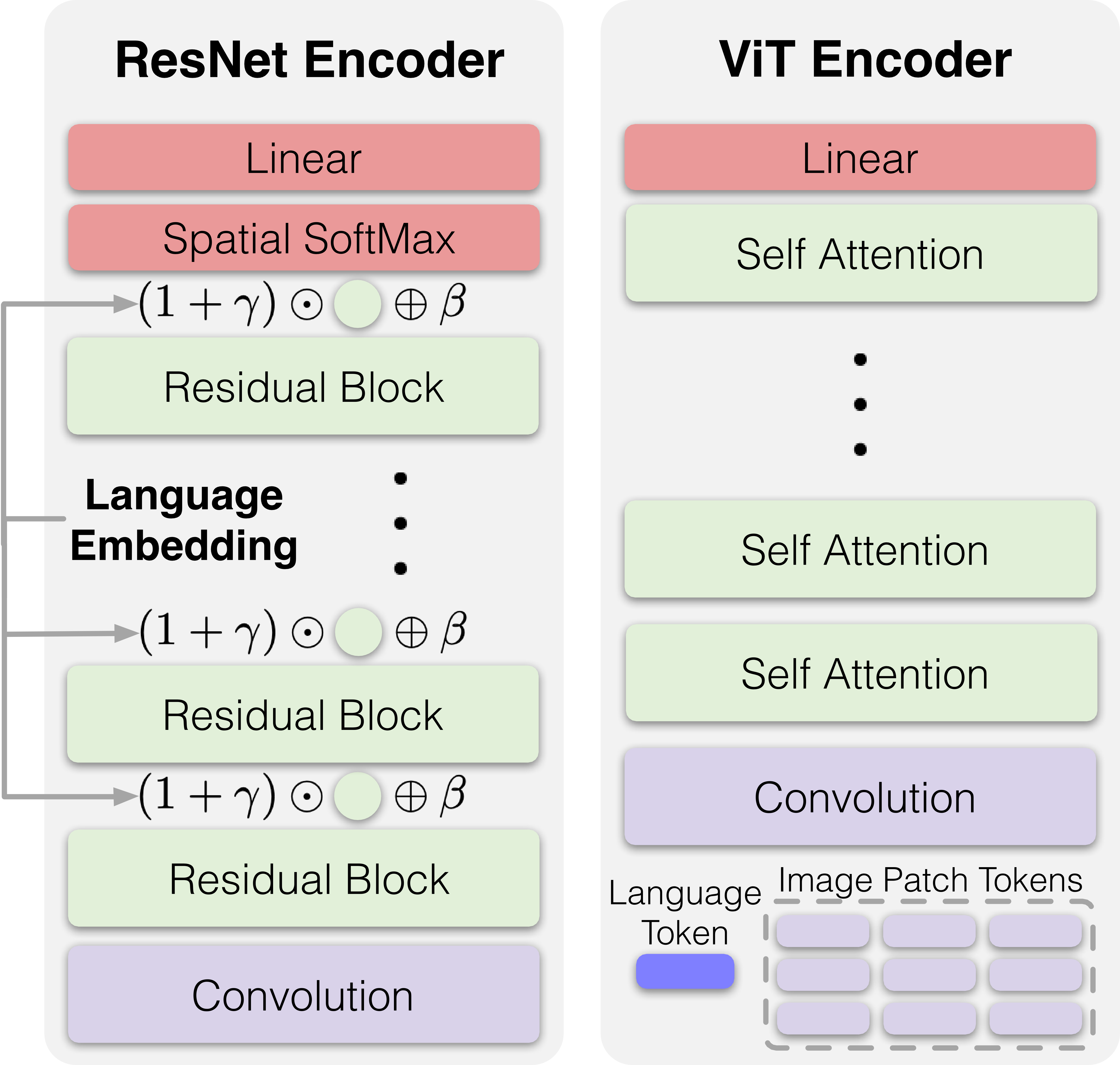
BCRNNPolicy (ResNet-LSTM)#
(See Robomimic)
The visual information is encoded using a ResNet-like architecture, then the temporal information is summarized by an LSTM. The sentence embedding of the task description is added to the network via the FiLM layer.
BCTransformerPolicy (ResNet-Transformer)#
(See VIOLA)
The visual information is encoded using a ResNet-like architecture, then the temporal information is encoded by a temporal transformer that uses the visual encoded representations as tokens. The sentence embedding of the task description is added to the network via the FiLM layer.
BCViLTPolicy (ViT-Transformer)#
(See VilT)
The visual information is encoded using a ViT-like architecture, where the images are patchified. Then the temporal information is summarized by another transformer. The sentence embedding of the task description is treated as a token into the spatial ViT.