Overview
Contents
Overview#
In the initial study of LIBERO, we conduct multiple experiments under the following five topics:
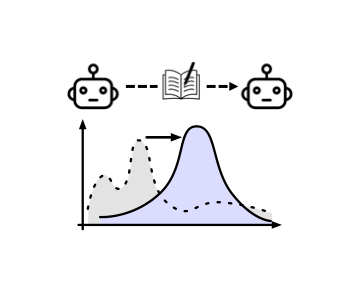
1. Knowledge Transfer under Specific Distribution Shift
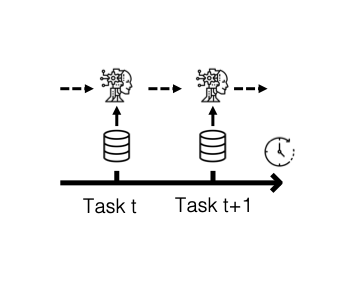
2. Design of Lifelong Learning Algorithm
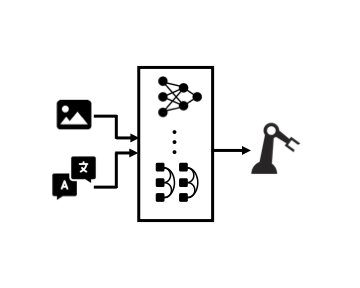
3. Design of Vision-Language Policy Architecture for Lifelong Learning
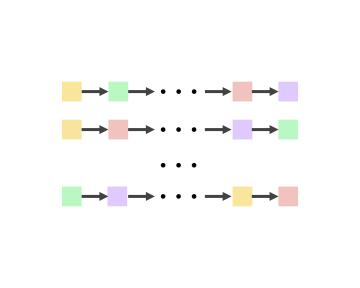
4. Robustness to Task Ordering
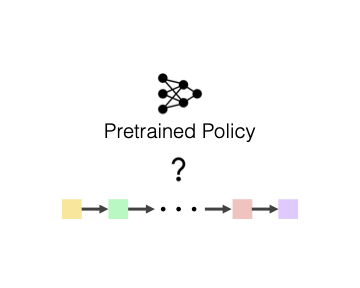
5. Effect of Pretraining on Lifelong Learning
Scripts for reproducing the results#
The lifelong/main.py
and lifelong/evaluate.py
scripts are the two main scripts used to reproduce our experiments. By default, main.py
performs both training and evaluation. However, if you have limited resources and want to separate the training and evaluation steps, you can turn off evaluation in main.py
and do the evaluation separately using evaluate.py
. To learn how to run the scripts, please read the pages under the Research Topic session.
Note: How to run LIBERO outside the repo?
If you want to use LIBERO as a separate module outside of the repository, we provide command scripts lifelong.main
and lifelong.evaluate
. Simply use them with the arguments you would use for the python scripts, and you can launch the experiments and save the results in your local project folder!
How to interpret / visualize the results?#
The result will be , by defautlt, saved into a folder named like experiments/LIBERO_90/SingleTask/BCViLTPolicy_seed100/run_001
, where the results are stored in this folder. The results consist of two parts: result.pt
and task{TASK_INDEX}_auc.log
. Both of them are saved through torch.save
function, so in order to read them out, you should use torch.load
function.
result.pt
saves the information regarding lifelong learning, which as the following structure: (TODO).
{
'L_conf_mat': np.zeros((NUM_TASKS, NUM_TASKS)), # loss confusion matrix
'S_conf_mat': np.zeros((NUM_TASKS, NUM_TASKS)), # success confusion matrix
'L_fwd' : np.zeros((NUM_TASKS,)), # loss AUC, how fast the agent learns
'S_fwd' : np.zeros((NUM_TASKS,)), # success AUC, how fast the agent succeeds
}
task{TASK_INDEX}_auc.log
saves the information regarding the training in single task, saving the success rates and the training loss every 5 epochs. More concretely, each .log
file contains the following information (the values are examples):
{
'success': array([0.08, 1. , 1. , 0.42, 1. , 1. , 0.98, 1. , 1. , 0.92, 0.98]),
'loss': array([ 5.43036654, -15.55810809, -17.16367282, -18.11807803,
-18.97084025, -19.48923949, -20.5096283 , -21.28826938,
-21.83023319, -22.32912602, -22.60586715])
}